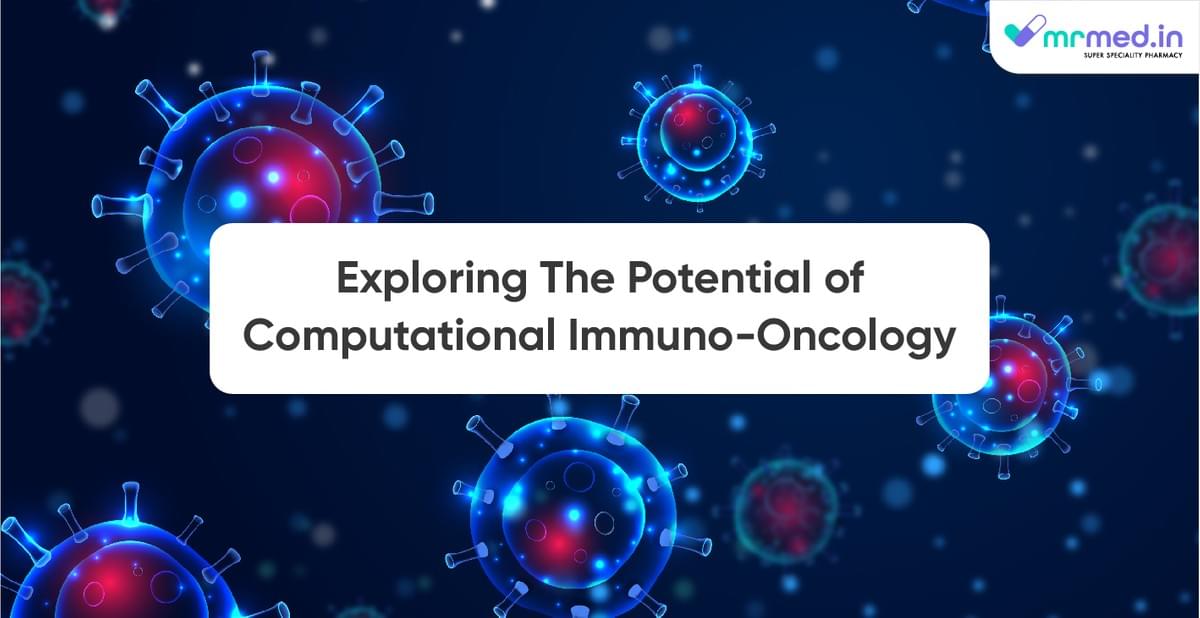
Cancer has been a formidable adversary in the realm of healthcare for decades, claiming millions of lives each year worldwide. While traditional treatments such as chemotherapy and radiation therapy have made significant progress in combating this complex disease, the field of oncology is undergoing a transformation. Computational immuno-oncology, a burgeoning discipline at the intersection of computer science, immunology, and oncology, holds the potential to revolutionise cancer treatment by harnessing the power of the immune system and cutting-edge computational technologies. In this article, we will delve into the promising realm of computational immuno-oncology, exploring its role in the fight against cancer and the transformative impact it could have on patient care.
Understanding the Immune System's Role in Cancer
To comprehend the significance of computational immuno-oncology, it is essential to grasp the pivotal role of the immune system in identifying and eliminating cancer cells. Our immune system is an intricate network of cells, tissues, and organs that work together to defend the body against foreign invaders, including cancer. Immune cells, such as T cells and natural killer cells, play a critical role in recognizing and attacking abnormal cells, including cancerous ones. However, cancer cells often evade detection or develop mechanisms to suppress the immune response.
This is where computational immuno-oncology steps in, leveraging advanced computational tools and techniques to decipher the complexities of the immune system's interaction with cancer and develop innovative strategies for boosting the body's natural defenses against the disease.
The Promise of Immunotherapy
Immunotherapy, a groundbreaking approach to cancer treatment, has gained significant attention in recent years. It focuses on enhancing the body's immune response to target and destroy cancer cells. Some of the most promising immunotherapies include checkpoint inhibitors, chimeric antigen receptor (CAR) T-cell therapy, and cancer vaccines.
Checkpoint inhibitors, for instance, block certain proteins that inhibit immune responses, allowing the immune system to recognize and attack cancer cells effectively. CAR T-cell therapy involves genetically modifying a patient's T cells to target specific cancer antigens, while cancer vaccines stimulate the immune system to recognize and attack cancer cells.
While immunotherapy has shown remarkable success in some cancer types, its effectiveness can vary widely from patient to patient. Computational immuno-oncology seeks to address this variability by tailoring treatments to individual patients based on their unique genetic and immune profiles.
Personalized Medicine in Cancer Treatment
Personalized medicine, a core principle of computational immuno-oncology, aims to deliver highly targeted and effective treatments by considering the individual characteristics of each patient's cancer. This approach recognizes that cancer is not a uniform disease, and that each patient's tumor is unique, requiring tailored therapies.
Advances in genomics and bioinformatics have enabled researchers to analyze a patient's DNA and identify specific genetic mutations or alterations driving their cancer. By understanding the genetic landscape of a patient's tumor, oncologists can select the most appropriate immunotherapy or combination of treatments, significantly increasing the likelihood of a successful outcome.
The Integration of Big Data
One of the driving forces behind computational immuno-oncology is the integration of big data. The field relies heavily on collecting, storing, and analyzing vast amounts of data, including genomic data, clinical records, and immune system profiles. These data are then processed using sophisticated computational algorithms and machine learning techniques to extract valuable insights.
For instance, researchers can identify biomarkers that predict a patient's response to immunotherapy or the likelihood of cancer recurrence. By analyzing real-world patient data, they can also refine treatment protocols and discover previously unrecognized patterns that inform treatment decisions.
Artificial Intelligence and Machine Learning
Artificial intelligence (AI) and machine learning (ML) are integral components of computational immuno-oncology. These technologies have the capacity to analyze complex datasets with unprecedented speed and accuracy, aiding in the discovery of novel drug targets and treatment strategies.
Machine learning algorithms can predict how individual patients will respond to specific treatments based on their genetic and clinical profiles, helping oncologists make more informed decisions about the most suitable therapies. Furthermore, AI-driven image analysis can improve the early detection of cancer by identifying subtle abnormalities in medical images, such as mammograms and CT scans.
Clinical Decision Support Systems
Computational immuno-oncology is also advancing the development of clinical decision support systems (CDSS), which assist healthcare providers in making treatment decisions. These systems integrate patient data, scientific research, and treatment guidelines to recommend the most appropriate therapies for individual patients.
CDSS can help oncologists navigate the complexity of cancer treatment options, especially in cases where multiple therapies are available, and each may have distinct risks and benefits. By providing evidence-based recommendations, CDSS can enhance the quality of care and potentially improve patient outcomes.
Challenges and Ethical Considerations
While computational immuno-oncology holds immense promise, it is not without its challenges and ethical considerations. The field relies heavily on the availability of high-quality data, which may not always be accessible, especially for rare cancer types. Privacy concerns surrounding the sharing of patient data also need to be addressed to facilitate collaborative research efforts.
Additionally, the cost of implementing computational immuno-oncology approaches and integrating them into clinical practice can be substantial. Ensuring equitable access to these cutting-edge treatments is a priority.
Furthermore, the ethical implications of using AI and machine learning in healthcare, including issues related to bias in algorithms and the potential for automation to replace human decision-making, require careful consideration.
Conclusion
Computational immuno-oncology represents a beacon of hope in the fight against cancer. By leveraging the power of computational technologies, genomics, and immunology, this emerging field is poised to revolutionize cancer treatment. The ability to personalize therapies based on individual patient characteristics, predict treatment outcomes, and develop novel immunotherapies offers new avenues for improving patient care and outcomes.
As computational immuno-oncology continues to evolve, it is essential to address challenges related to data access, privacy, cost, and ethics. Collaborative efforts among researchers, clinicians, and policymakers are crucial to realizing the full potential of this transformative field and ensuring that the benefits of computational immuno-oncology are accessible to all those in need.
In the years to come, computational immuno-oncology is likely to play an increasingly central role in the fight against cancer, offering hope to patients and advancing our understanding of this complex and devastating disease.